Research study:
Information design in public transportation
2019Extract from my research done in 2019.
You can read the complete research on Medium
1 - The story behing Paris’ transit map
2 - Analysis of travelling behaviour
3 - Research insights
“92 minutes is the average time people in the Paris area spend on public transport. As transportation infrastructure is pushed to its limit, demand for an alternative means of transport has emerged and pressure to optimize the existing system continues to grow.
Schematic diagrams are a popular and efficient way to map the transit system of urban cities. However, with the increase in the number of stations and the rapid development of infrastructure, it got me wondering if this representation is still up-to-date and if it is the best way to help passengers navigate.
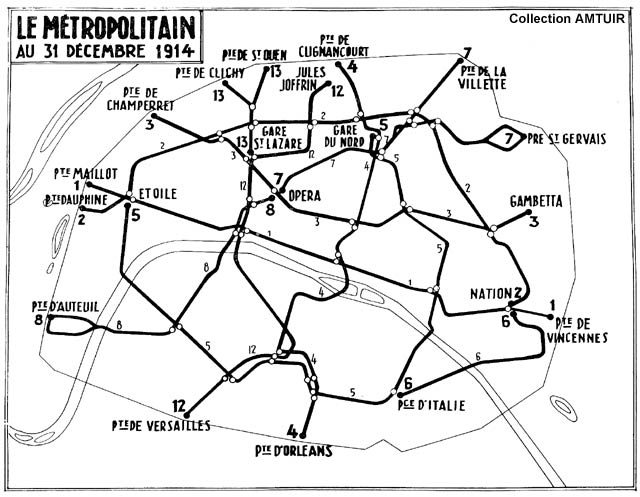
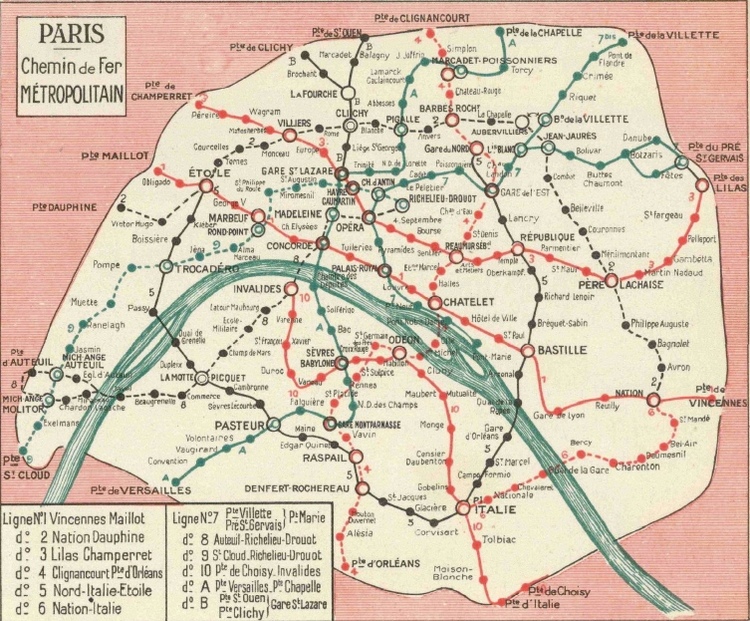
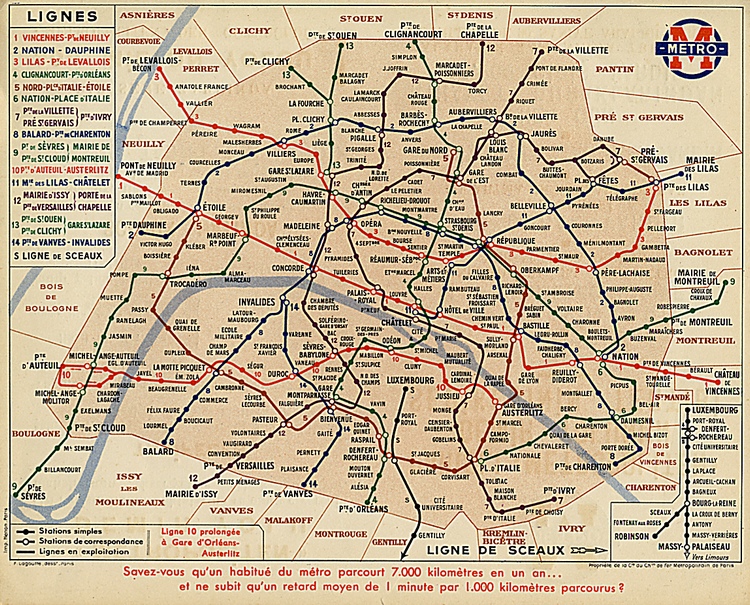
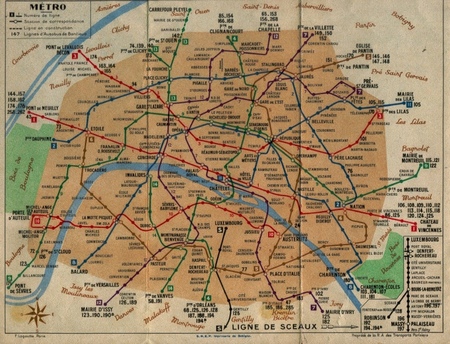
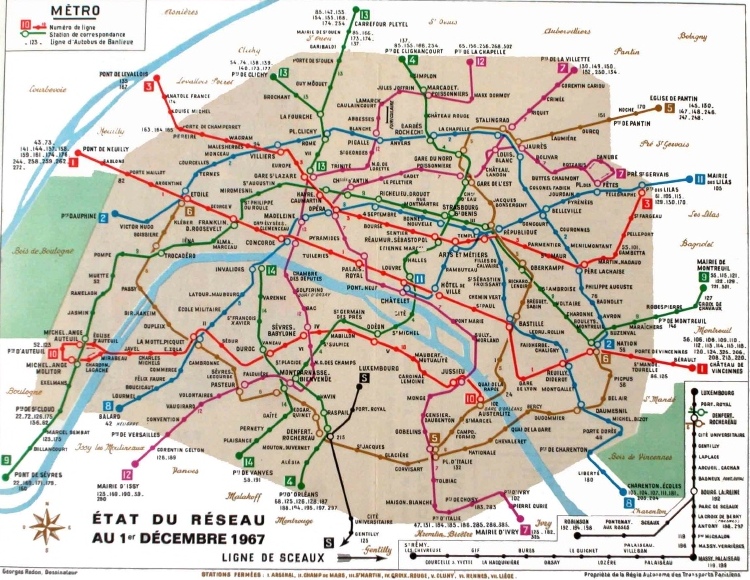
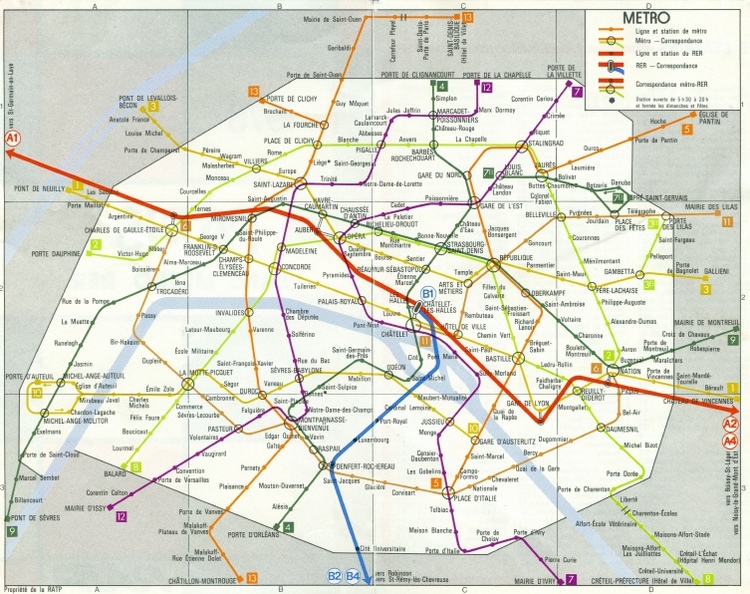
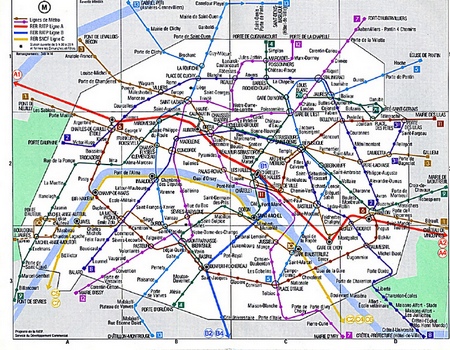
From left to right, top to bottom: Paris’ schematic map in 1914, 1930, 1939, 1967, 1978,
How might we better use transit maps to encourage passengers to use public transportation in the most convenient way?
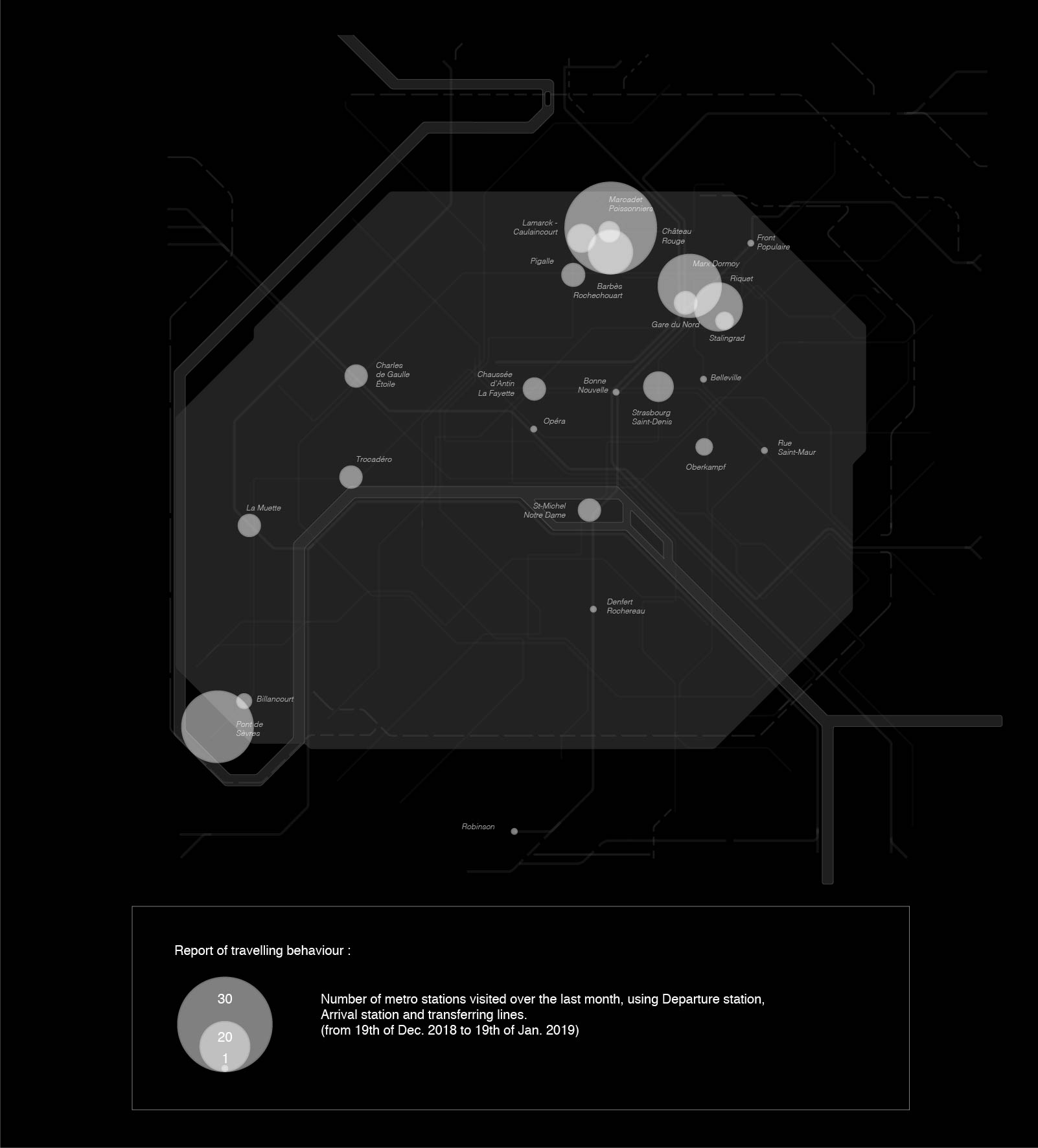
Assumption 1: People only use a limited number of stations in the entire network.
During one month, I reported every single path I took with the sequence of departure stations, transfer stations and arrival stations (D-A pairs). If I was walking or biking, I just reported the closest metro station.
During one month, I reported every single path I took with the sequence of departure stations, transfer stations and arrival stations (D-A pairs). If I was walking or biking, I just reported the closest metro station.
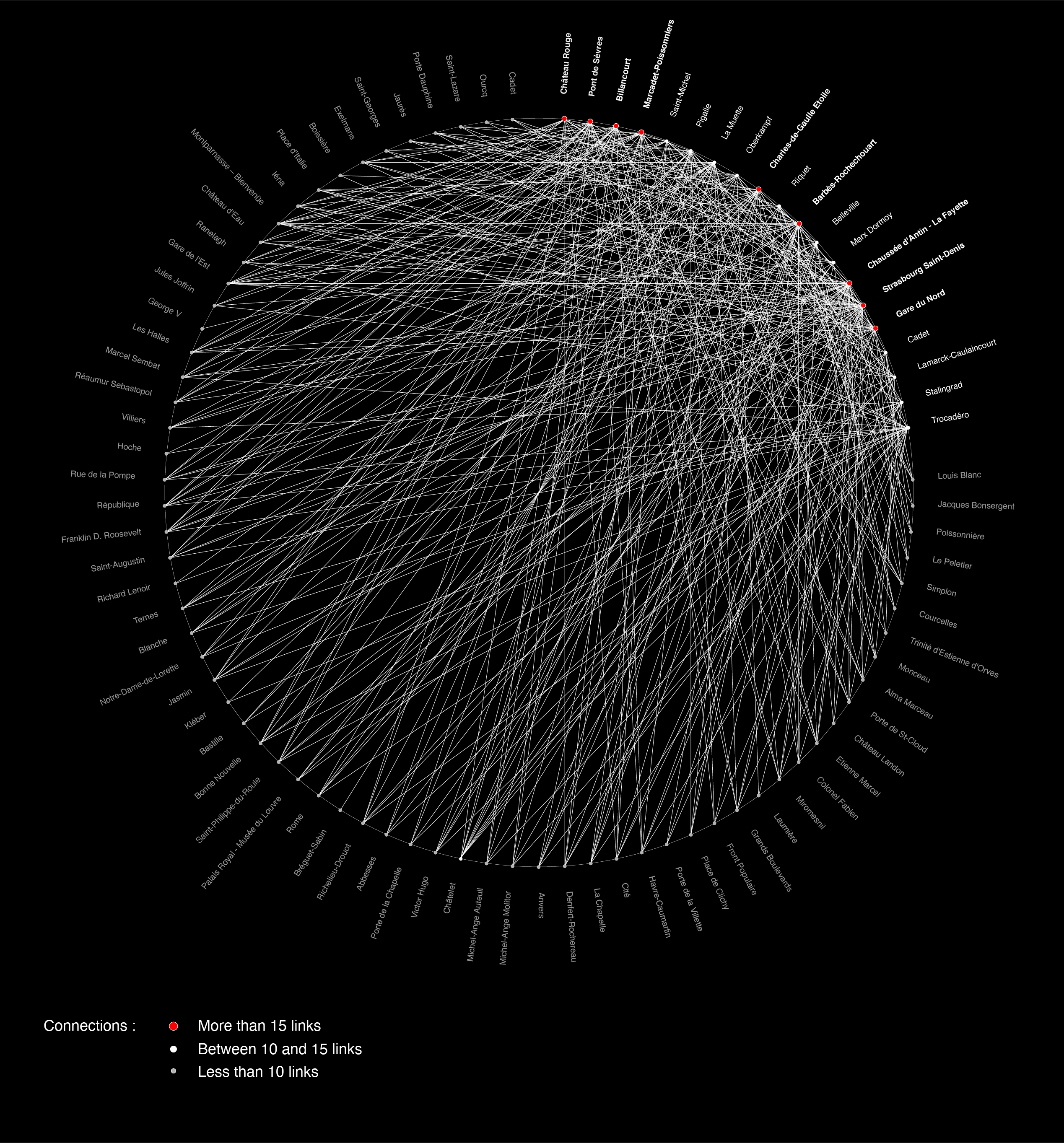
Assumption 2: Some stations are overly full and others are completely empty.
In the diagram below you can see the different stations I used according to my travelling report. The top left stations are my most 20 visited stations whereas the other ones in the circle represent the stations I have to go through to reach the final destination. By matching my most visited stations with the other ones on the lines they belong to, a network of connections emerged.